Artificial Intelligence (AI) has evolved from a science fiction concept into an integral part of our digital lives. One pivotal development in this regard is generative AI – a revolutionary technology that pushes the boundaries of what machines can create. This article uncovers the core aspects of generative AI, its influence on various industries, and the ethical concerns it raises, and anticipates what the future holds for this groundbreaking technology.
What Is Generative AI Technology?
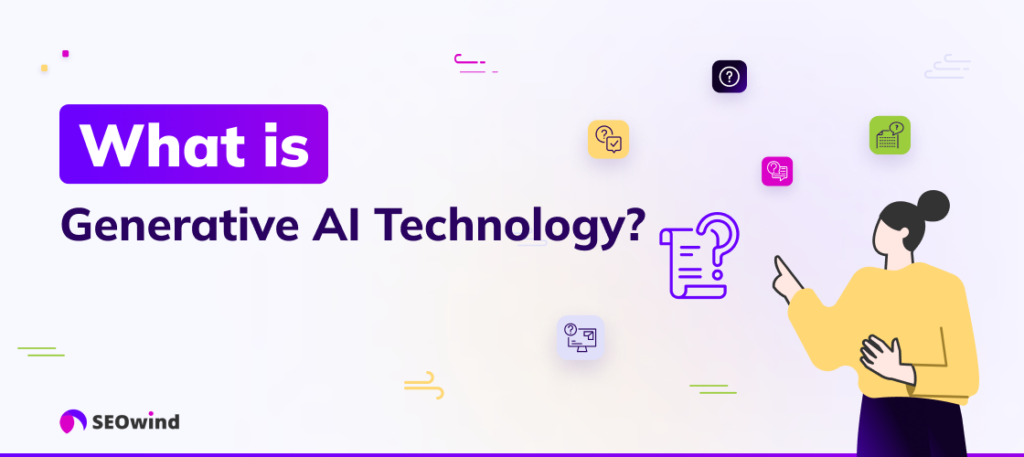
Generative AI, among the types of AI models, leverages algorithms to produce outputs from scratch. It differs distinctly from other machine learning methods by creating new data instances similar to a provided set. The output may incorporate images, music, or text and often astounds with its likeness to human-created content or natural language.
What is generative AI vs machine learning?
Machine learning is a subset within the larger domain of artificial intelligence. Its primary focus involves teaching machines to learn patterns from existing data and making predictions or decisions without explicit programming. On the contrary, while generative AI utilizes pattern recognition, its key characteristic is generating novel data that simulate a specified style or format based on acquired knowledge.
Contrary to regular machine learning models with capabilities focused more on comprehension and forecasting tendencies in input data, generative AI steps further into creativity’s realm using techniques such as Google’s ‘generative adversarial networks’ (GAN).
What is the difference between generative AI and AI?
Artificial Intelligence is an umbrella term covering a broad spectrum of technologies enabling computers to mimic human intelligence’s basics – understanding, reasoning, and problem-solving, among others.
Generative Artificial Intelligence represents a class within these technologies focusing on creation or generation abilities simulating human-like creativity. This distinct aspect makes it different but not exclusive from other branches under the grand canopy termed ‘Artificial Intelligence’ like net AI or predictive AI.
What Are the Benefits of Generative AI?
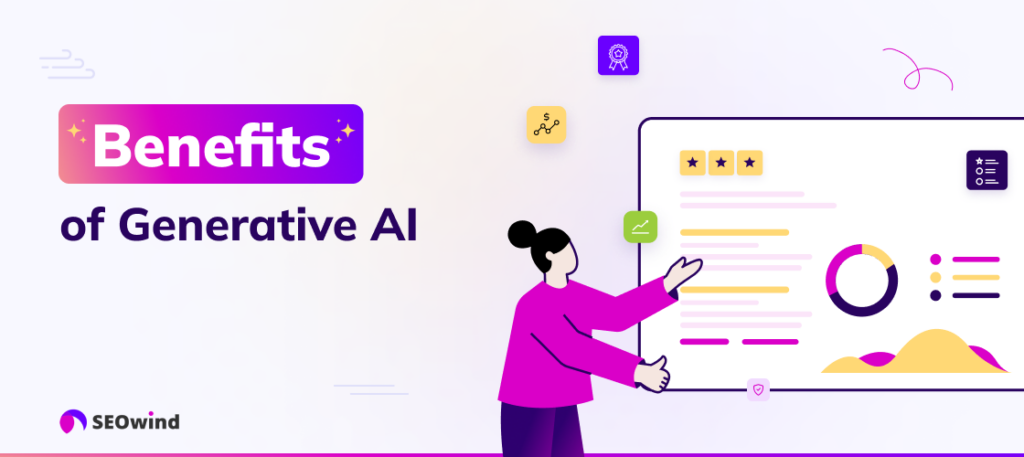
Generative AI, a subtype of artificial intelligence generation, holds remarkable benefits with its transformative potential. While it pushes the boundaries of creativity and innovation, let’s delve into the intricacies of what this impressive technology opens up for us.
- Expeditious Productivity: With generative AI tools at our disposal, the speed and efficiency of tasks can experience a boost manifold. These tools can generate designs, text, or even music much faster than human counterparts without tiring.
- Efficient Data Usage: Google generative AI models are built leveraging large volumes of AI training data that is used cleverly to extract patterns and insights, which in turn aids in generating new content.
- Predictive Strength: The use of generative AI vs predictive AI may trigger specific questions, but here’s how the former stands out—it learns from existing data and predicts novel outputs, unlike predictive AI, which only forecasts based purely on past trends.
- Infinite Creativity Pool: From generative text creation to making unique designs, generative AI examples are plentiful and testify to its creative prowess. It offers an infinite pool of originality previously impossible for standard machine-learning technologies.
- Cost-Effective Solutions: There’s no denying that tasks involving repetition can be efficiently handled by AI-generated models, thereby saving substantial operational costs while improving process accuracy.
In essence, generative AI hails as a game-changer with capabilities beyond usual machine learning solutions or regular net AI paradigms and possesses endless possibilities waiting to be unlocked across various fields.
What Are the Limitations of Generative AI?
As impressive as generative AI may seem, several limitations are worth noting with this advanced AI model. Herein, I explore some of these complexities and restrictions:
- Dependence on Quality Training Data: Like many other categories in artificial intelligence, generative AI is highly dependent on training data. However, securing high-quality datasets can be a difficult challenge. If the dataset needs to be more diverse and representative, the capability and efficiency of the generative models can be significantly limited.
- Risk of Generating Unethical Content: It should also be noted that generative AI has the potential to create content that could violate ethical standards or even propagate harm. This largely stems from its ability to replicate and misuse sensitive information, part of its training data.
- Need for Greater Computer Resources: Generative models require large amounts of computational resources — far more than traditional predictive AI tools do.
- Opaque Decision-Making: In some instances, interpreting how exactly a generative model came up with its output can become quite an arduous task—a complication commonly referred to as ‘AI opacity.’
With thoughtful consideration and carefully designed implementations, we stand poised at the precipice of a future where generative technology will unquestionably play a prominent role across sectors – influencing productivity, design processes, and more.
The technological hurdles we currently perceive as limitations are merely stepping stones in our evolving understanding and utilization of this transformative type of AI model—Generative AI.
Next on our list, let’s dive into some ethical considerations surrounding using AI applications like generative AI— from privacy concerns to issues around bias inherent in Ai-generated models.
What Are the Concerns Surrounding Generative AI?
While exploring Generative AI, its capabilities, and its influence, it’s essential not to overlook some of the critical concerns surrounding this revolutionary technology. Despite the multiple advantages Generative AI delivers, their potential misuse and several fundamental limitations call for prudent caution.
Human authorship
Firstly, there is growing concern regarding how generative text created by sophisticated tools can blur the line between machine-generated content and human authorship. As an example, ChatGPT proves that machines can now produce high-quality prose indistinguishable from human-written texts invalidating the phrase ‘seeing is believing.’ This ability may pave the way for potential misinformation campaigns or the creation of fake news items, significantly disrupting our information-dependent world.
Data privacy
Secondly, data privacy considerations arise as with many AI models, including Google’s Generative AI project known as Magenta. Personal user data is often used in training these models. The question here revolves around user consent and safety: Is enough being done to adequately inform users about how their own data is used? Could potentially sensitive information inadvertently be incorporated into the outputs from a generatively trained model?
Bias
Thirdly, there’s concern about bias within generative AI models created by net AI and others. A stark reminder that these powerful tools are only as unbiased as their training data allows them to be. Inherent biases in datasets might unknowingly reproduce discriminatory behavior when deployed on a broader scale-often called ‘algorithmic bias.’
Job losses
Lastly, many fear widespread deployment of advanced models like Generative AI would lead to job losses, particularly within creative sectors such as writing and design.
Delving deeper into this topic will help us better comprehend what generative AI entails and maybe even ascertain some solutions moving forward. With rapid advancements in this field fuelled by increased automation needs worldwide, together with enormous strides made in artificial intelligence generation technologies in recent times—it seems inevitable that we’ll have to confront these issues sooner rather than later.
What Are Some Examples of Generative AI Tools?
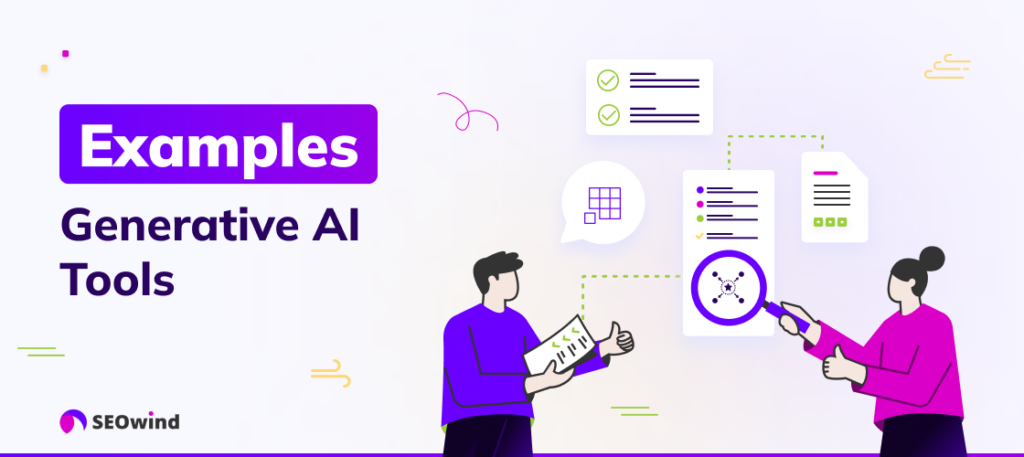
Today, multiple examples of generative AI tools made significant marks in various industries ranging from art, gaming to even healthcare. These sophisticated software programs precisely carry out tasks and create effective solutions that simulate human-like activities.
One such example is DeepArt, an online platform using generative AI capabilities for transforming user-uploaded pictures into digital artwork in the style of famous artists. Leveraging the power of “generative,” it restructures your images not by applying filter overlays but by understanding artistic nuances and recreating them into realistic images.
Similarly, the development community was recently taken by storm with the emergence of ChatGPT – another excellent example of a generative AI tool produced by OpenAI. This artificially intelligent chatbot uses machine learning algorithms to mimic conversations with human users, improving how we use and perceive automated customer service bots.
This list includes Google’s Magenta project, explicitly designed to push boundaries generatively within music creation. Its primary role is producing original compositions based on specified parameters set by the user giving rise to unique creations unprecedented in the traditional musical landscape.
Also noteworthy are GANPaint Studio, a research experiment from MIT making image editing more intuitive through its deep learning model, and NVIDIA’s GauGAN, a web app painting simulator coalescing neural network-powered image interpretation systems making scene generation seamless.
Lastly, Runway ML, an everyday tool for creatives, interacts primarily with design models translating abstract concepts into visually aesthetic outcomes.
These tools illustrate some applications and capabilities for which generatively trained data models are designed. Each opens up new avenues for automation, fostering creativity while raising thought-provoking questions about ‘artificial’ intelligence invading traditional ‘human’ domains. However you look at it, one thing is clear: The concept of generative AI continues to reshape our technological future.
Use Cases for Generative AI by Industry
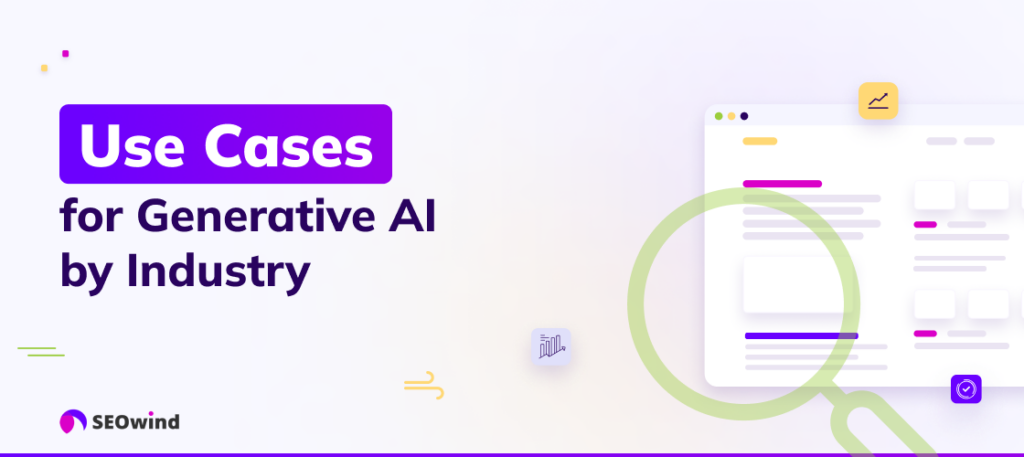
Generative AI is fostering significant advancements in various industries. It’s essential to explore the influence and power of these generative AI tools within different sectors and understand how businesses can benefit.
Healthcare Sector
The healthcare sector has been significantly enriched with the aid of generative AI. The technology’s ability to examine patient data, develop potential diagnoses, and generate treatment options makes it invaluable. Genomics research, drug discovery, and biomarker development are areas where generative models are extensively used.
Marketing & Advertising
In marketing and advertising, creativity is king. Fortunately, that’s one thing generative AI excels at. From creating engaging ad content to developing unique marketing strategies – every step becomes streamlined with these ai-generated models. Google generative AI has developed some fascinating instances of auto-ad creation fueled by complex data and by deep learning technologies.
Manufacturing
These AI models also play a crucial role in manufacturing, with applications ranging from designing complex parts using 3D printing to predicting failures based on existing patterns. Generative AI could be the game-changer in quality control techniques, workflow optimization, and Design for Manufacturing (DFM).
Entertainment
It wouldn’t be amiss to say that entertainment – music, gaming, film production – has experienced a revolution due to advances in generative technology. One example includes chatGPT generative AI, used to create dialogue for video games or movies.
This roundup only scratches what can be achieved using Ai tools like Generative Models across industries. As more companies leverage this exciting technology for innovation and growth, the future may reveal many such endeavors where gen ail influences industry performances in unimaginable ways.
Ethics and Bias in Generative AI.
The expanding applications of generative AI naturally invite a range of pertinent ethical considerations. Notably, integrating biases into AI models, often called ‘algorithmic bias,’ forms an integral part of this discourse.
Algorithmic Bias: A Side Effect of Generative AI?
Generative AI relies heavily on training data for its functionality. This process, known as “AI training,” is where biases typically get introduced. The resulting generative model will likely perpetuate those inclinations if the input data embodies certain prejudices or biases.
It implies that despite their technological sophistication, these systems have inherited human flaws and, thus, might not be entirely objective or fair. For instance, concerns abound about job application screening tools favoring candidates based on race or gender due to biased algorithms.
The Impact of Biased Training Data
To further illustrate this point, consider a popular type of model used in generative text applications – Google’s generative AI has produced phenomenal works thanks to its high-quality training data.
However, if misused or fed with skewed data, it could churn out harmful content. In essence, algorithmic ethics are paramount when dealing with various forms like chatGPT generative AI or other Ai models.
Addressing Ethical Challenges in Generative AI.
Addressing these issues requires a rigorous examination of the training datasets utilized by these Ai generations. Additionally,
- Measures should include regular audits aiming at minimizing prejudice within modeling processes.
- Emphasizing building more diverse sets of training data can help limit bias.
- Organizations need transparency regarding how AI determines decisions.
- Encourage interdisciplinary collaboration between technologists and ethicists during generative model development.
In conclusion, creating unbiased Ai-generated models isn’t beyond reach, but it surely needs our continued attention and effort toward promoting a healthier relationship with generative technology.
Generative AI vs Artificial Intelligence
Now that we’ve somewhat demystified the concept of generative AI, it’s imperative to talk about how it stands in contrast with its parent field, artificial intelligence (AI). This section will provide valuable insights if you’re among those asking ‘What is gen AI?’ or trying to learn ‘How is AI trained?’.
Artificial Intelligence involves various tools and models that enable machines to mimic human thinking or learning patterns. The spectrum of AI includes different types, including the ‘reactive,’ ‘limited memory’ intelligence, right up to self-awareness stages. In comparison, generative AI represents a specific strand within the vast network of AI model types.
So where do these two diverge? Think about traditional artificial intelligence as reactive; systems can understand what is required from them and respond accordingly, but they lack creativity or innovation. They cannot generate anything new on their own. But here’s where generative artificial intelligence kicks in – it appears transformative by nature due to its ability to create novel outputs.
With a central focus on using algorithms to generate creative outcomes such as images, music, and text from scratch—generative models constitute the fuel behind most exciting “AI-generated” content today: think Google’s DeepDream for creating surreal images or OpenAI’s ChatGPT for crafting full-length articles like humans do! Thus, this distinctive feature sets generative AI apart from conventional artificial intelligence.
Yet some similarities bind them together just as powerfully as their differences set them apart. Both deployments of technologies thrive on rigorous “ai training data.” From utilizing pre-existing datasets derived from human users or simulations, these evolving systems absorb information and strive towards optimizing performance over time.
In summary, though distinctively different at a functional level, both disciplines underpin machine intelligence’s strength and increasingly pervasive presence today.
Generative AI History
The history of generative AI is a riveting tale, marked by calculated steps toward advancement and innovation. From its inception to present-day accomplishments, this exciting field has experienced significant evolution.
In the early stages, researchers sought to decipher what makes a generative model work in artificial intelligence. I would classify this as the first ‘generation’ in generative AI’s journey—a period of defining the foundation model and understanding its core mechanisms.
In 2006, Geoffrey Hinton introduced Deep Belief Networks (DBNs), propagating an era of deep learning models. These were attempts at using neural networks to generate new data resembling inputs provided during training—ushering in the second generation.
Later, around 2014, Ian Goodfellow et al. contrived Generative Adversarial Networks (GANs). These distinctive algorithms employed two neural network models competing against each other: one generating samples and another evaluating them—a monumental progression that arguably characterizes the third ‘generation.’
It’s essential also to mention Googlegoogle’s generative ai contributions during these periods, such as the DeepDream art project, which transformed our perception of AI capabilities. This was all made possible due to advancements in neural net architectures and larger-scale implementations empowered by increased computing power and more diverse training data sets.
Alongside these developments occurred those with ChatGPT generative AI – OpenAI’s transformer-based language processing software famous for its human-like text production capabilities – further validating the potential of generative models.
As we continue this discovery, generative AI’s exploration and innovation path remains ceaseless. New frameworks and types of AI models continue to emerge, often serendipitously from previous works like GANs or DBNs, continually fine-tuning our collective comprehension of how this dynamic technology can be harnessed for more profound applications.
Understanding the Functioning of Generative AI Models
Generative AI models, at their core, are designed to understand and replicate patterns, whether these are in words, images, sounds, or other forms of data. Their primary aim is to generate new data that can pass as genuine or original, even though it’s machine-generated.
Machine learning and neural networks
To comprehend the functioning process of a generative AI model, it is essential to delve into its fundamental bases: machine learning and neural networks. Machine learning algorithms teach the system how to learn from data inputs and use this learned information to make predictions or decisions rather than being specifically programmed to carry out a task. A particular type of machine learning model responsible for the generative properties of AI is the artificial neural network (ANN), which attempts to simulate the functionality of human neural networks.
Generative Adversarial Networks (GANs)
Deep learning, a subset of machine learning, leverages complex neural networks called Generative Adversarial Networks (GANs). GANs consist of two vital components: the generator and the discriminator. These two are engaged in a continuous game of cat and mouse, where the generator aims to produce artificial outputs, and the discriminator attempts to distinguish the counterfeit from the real data.
In a standard training cycle of a GAN, the generator will start by generating some initial output based on random data – let’s say an image. The discriminator will then learn to classify this image as false. As the training continues, the generator gets better at creating images that are increasingly similar to the real ones. At the same time, the discriminator becomes adept at identifying which images were created by the generator.
The model’s training continues until the discriminator can no longer reliably tell whether the images are from real world images or fictitious. The generator aims to produce such close replicas of the desired output that the discriminator (and, by extension, we humans) cannot distinguish them from the original data.
Generative AI models have become the underpinning technology for creating realistic artificial voices, creating art, synthesizing images, generating text, and even creating autonomous agents in video games. They have immense potential in their applications, paving the way for technological breakthroughs that can significantly impact areas like design, entertainment, healthcare, and even our day-to-day lives.
Best Practices for Using Generative AI.
As we delve into generative AI, it’s crucial to discover and deploy the best practices. These guidelines will assist in utilizing this potent tool effectively while mitigating potential risks.
Understanding Data Requirements
Generative AI training demands vast amounts of data. Since these AI-generated models derive knowledge from previously fed information, understanding what data you need is paramount. Quality takes precedence over quantity; providing erroneous or irrelevant details can lead to counterproductive results.
Constant Monitoring and Updates
Generative AI could grow obsolete without regular upgrades and updates like any other technology. Staying up-to-date with the latest Generative AI news and trends helps optimize operations. Additionally, constant monitoring can detect anomalies early on, allowing prompt solutions before they cause colossal impact.
Consider Ethical Implications
Although beneficial, generative AI does pose concerns surrounding ethics. As a user of such advanced technology, one must ensure its ethical use – not violating privacy rights or unintentionally propagating bias.
- Be aware of biased input data: Amidst providing Ai training data, watch out for any biases that could tarnish the final output.
- Respect User Privacy: With burgeoning discussions around how AI is trained to echo privacy questions, Respecting regulations is essential to maintain consumer trust.
- Do not use it maliciously: Assets like ChatGPT generative Ai bear immense power that might be misused in forging documents or creating deceptive content.
Knowing When Not To Use It
Just because “What is gen ai” showcases an array of applications doesn’t mean every scenario calls for it. Identifying when not to deploy generative text or tools requires discernment and experience.
In conclusion, being adept at using Generative Ai is more than just employing the tool but also comprehending its workings thoroughly enough to recognize associated benefits & limitations. Adhering meticulously to these best practices when dealing with generative Ai will pave the way toward more efficient utilization and sustained progress within your business functions and industry domain.
The Future of Generative AI.
Looking toward the future, we can envision a world where generative AI plays an essential role. As the potential applications for this advanced technology continue to unfold, it’s clear that it will have a profound impact across various sectors.
The ongoing evolution of generative AI is likely to lead to some genuinely transformative developments. Here are some predictions about what the future might hold:
- Customized Content Creation: One astonishing capability of generative models is content creation. Tools like chatGPT generative AI already generate human-like text based on prompts. Over time, these tools will refine their abilities, delivering more customized and complex content with less human input required.
- AI-Generated Models Advancement: I anticipate remarkable enhancements in the accuracy and realism of AI-generated models. More nuanced 3D animations, photorealistic images from descriptions, or even creating detailed virtual environments could become routine tasks for advanced versions of today’s generative AI tools.
- Interactive Virtual Assistants: With advancements in speech recognition and comprehension capabilities, there’s no doubt that more sophisticated interactive virtual assistants are on the horizon. These AI-powered helpers will provide us with seamless conversational experiences and understand our needs better than ever before.
- Scientific Research & Innovation: Generative AI has tremendous potential to streamline scientific research. It could be used to create hypothesis-generating models, potentially accelerating breakthroughs in medicine and environmental science.
In conclusion, our journey toward fully understanding and harnessing artificial intelligence remains an ongoing endeavor full of excitement and challenges. The vast possibilities offered by generative AI imply that we’re at the cusp of a new era powered by boundless digital imagination!
AI Existential Risk: Is AI a Threat to Humanity?
Ever pondered the question, “Is AI a threat to humanity?” Indeed, it’s a popular topic for debate, with equally compelling arguments from both sides. Although generative AI presents many benefits and opportunities across multiple industries, concerns about its potential risks have begun to surface.
For instance, generative models could be leveraged by cybercriminals. With their capability to replicate human behavior convincingly, they can fabricate realistic phishing emails or fraudulent content—ultimately causing significant harm.
What further accentuates these concerns is that the sophistication of such tools keeps increasing. For example, ChatGPT Generative AI by OpenAI, an entirely text-based artificial conversational entity, can produce astonishingly ‘human-like’ dialogues. Its ability suggests possibilities of malicious exploitation if it falls into evil hands.
While this seems alarming, one must remember that any technology carries inherent risks along with its advantages. The key lies in establishing robust measures that mitigate possible misuse while enjoying the benefits offered by generative AI systems.
Equally important is an ongoing dialogue on ethics and bias in generative AI development—a significant step towards ensuring the responsible use of this powerful technology.
Academics across disciplines are working diligently on solutions so as not to let such an existential risk occur. Researchers keep pushing boundaries while also respecting ethical guidelines and exemplary practices.
Consequently, maintaining this delicate balance between technological advancement and ethical considerations is the primary challenge. As we continue exploring and evolving our understanding of Generative AI applications, their influence on society will undeniably remain an area requiring close monitoring and regulation.
Latest Generative AI News and Trends
Let’s delve into the most recent updates about generative AI as it continues to create a buzz in the technology industry due to its breakthrough capabilities.
Firstly, one cannot overlook OpenAI’s ChatGPT concerning generative AI tools. This natural language processing model has recently showcased advanced capabilities that capture users’ imaginations across various platforms.
Additionally, Google’s generative AI experiments are also making headlines. The tech giant is pushing boundaries by pioneering research in creative applications of these models, including artistry and music generation. These strides have heralded a new era where artificial intelligence doesn’t just analyze but creates content.
Notably, there are constantly emerging developments in how generative models can be trained more effectively upon diverse AI models, increasingly using real-world data for improved simulated outcomes.
Increasingly we’re also seeing a trend toward ethical considerations around using AI-generated models. While the benefits remain undeniable – from generating written content or designing webpages – growing concerns regarding authenticity and intellectual property rights are leading to active discussions on regulations.
The rising concern about bias within generative algorithms is inextricably tied to these ethical questions. This issue experts argue must be addressed urgently as further advancements in this field continue at an unparalleled pace.
While keeping track of specific trends may seem overwhelming given how rapidly they evolve, staying up-to-date will ensure you steer clear of outdated practices and harness the total capacity of this exciting component within AI generations!
What are some generative models for natural language processing?
Natural language processing (NLP) has remarkably advanced with the advent of generative models. These methodologies can generate new content by learning from provided data, making these models invaluable tools in various applications ranging from chatbots to automatic text summarization, translation, and much more. Here we delve into some of the most influential generative models for NLP.
Recurrent Neural Networks (RNNs)
The pioneering generative model for NLP, RNNs efficiently model sequences by maintaining a ‘hidden state’ from previous sequence steps. This state acts as a form of memory, influencing the current output and the future state. Although marred by the quandary of vanishing gradients, RNNs are instrumental in many foundational NLP applications.
Long Short-Term Memory Networks (LSTMs)
A particular kind of RNN, LSTM networks, mitigate the vanishing gradient problem with their unique cell state and gating mechanisms. LSTMs maintain and manipulate information for elongated periods, improving performance on tasks demanding long-term dependencies, like text generation or translation.
Gated Recurrent Units (GRUs)
Similar to LSTMs, GRU models avoid the vanishing gradient peril with gating mechanisms but achieve this with a simpler architecture. GRUs are a worthwhile alternative to LSTMs, particularly for tasks where computational efficiency is paramount.
Transformers
Initially introduced in the now-iconic “Attention is All You Need” paper, Transformers utilize self-attention mechanisms for considering the relevance of each word in a sequence when generating a new word. This allows the model to handle long-range dependencies and parallel computation, which is not feasible with RNNs.
Generative Pre-trained Transformer (GPT)
Built on the transformer model, GPT and its latest version, GPT -3, stand at the cutting edge of generative NLP models. Trained on vast quantities of text data, GPTs learn to predict the next word in a sentence, thus enabling text generation that can be astonishingly coherent, diverse, and contextually informed. GPT-3, the latest model boasting 175 billion machine learning parameters, represents the state-of-the-art in many NLP tasks and applications.
Bidirectional Encoder Representations from Transformers (BERT)
Another transformative model in NLP, BERT, differentiates itself by focusing on the context from both directions, bidirectionally, rather than just predicting the next word in a sequence. Although it is primarily used for NLP tasks like question answering or sentiment analysis, when fine-tuned, BERT can also serve as a generative model.
Seq2Seq Architectures
Seq2Seq models, comprising an encoder and a decoder component, are applied in tasks where input and output sequences have different lengths, like machine translation and speech recognition. While traditionally built on RNNs, LSTMs or GRUs, the advent of transformers has shifted the tides towards transformer-based Seq2Seq models, which exhibit superior performance.
While this list is not exhaustive, these models reflect generative tools’ growing power and sophistication in natural language processing. They have swiftly transformed NLP from a field of rigid rules and limited applications into one of creative potential, playing a fundamental role in the evolution of AI technology.